Scaling Smart in the Age of AI Agents: What We Learned at Startup Grind 2025
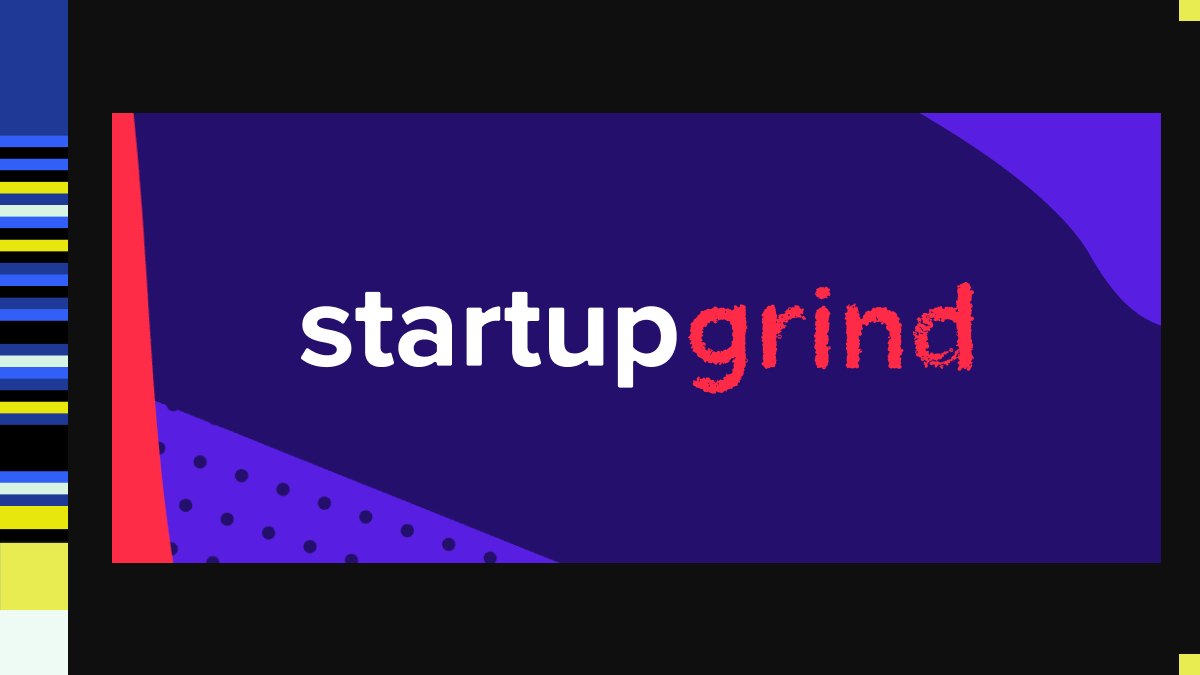
This April, founders, investors and operators gathered at Startup Grind Conference 2025 in Silicon Valley to talk about what’s working, what’s not and what’s next in an era where artificial intelligence adoption is rapidly picking up pace.
Brightwave Founder and CEO Mike Conover took the stage to share how AI agents are transforming high-stakes finance workflows like deal room due diligence and fundamental equity research. The real story, however, wasn’t just about the product’s evolution and capabilities. It was about mindset: what it means to build a truly AI-native company from day one.
Here’s what we took away from Mike’s talk, and from the broader Startup Grind experience.
From Tools to Agents: A Shift in Thinking
Most AI systems today still operate at the level of task automation. The next step, embodied by agentic systems, is the more challenging task of replicating the structure of human reasoning. Agentic AI doesn’t just summarize; it can process complex information, draw inferences and synthesize findings in a way that mirrors the analytical workflows of experienced professionals. Rather than executing isolated commands, agentic AI can form and refine hypotheses, test assumptions and build a coherent narrative from disparate data sources.
This shift from automation to reasoning marks a foundational change in how AI can be leveraged within complex workflows. AI now has the potential to no longer be just a bolt-on productivity tool but part of the decision-making infrastructure, enabling teams to operate with far greater scale, speed and analytical depth than traditional approaches allow.
The Latency Trap: Performance Isn’t Just About Speed
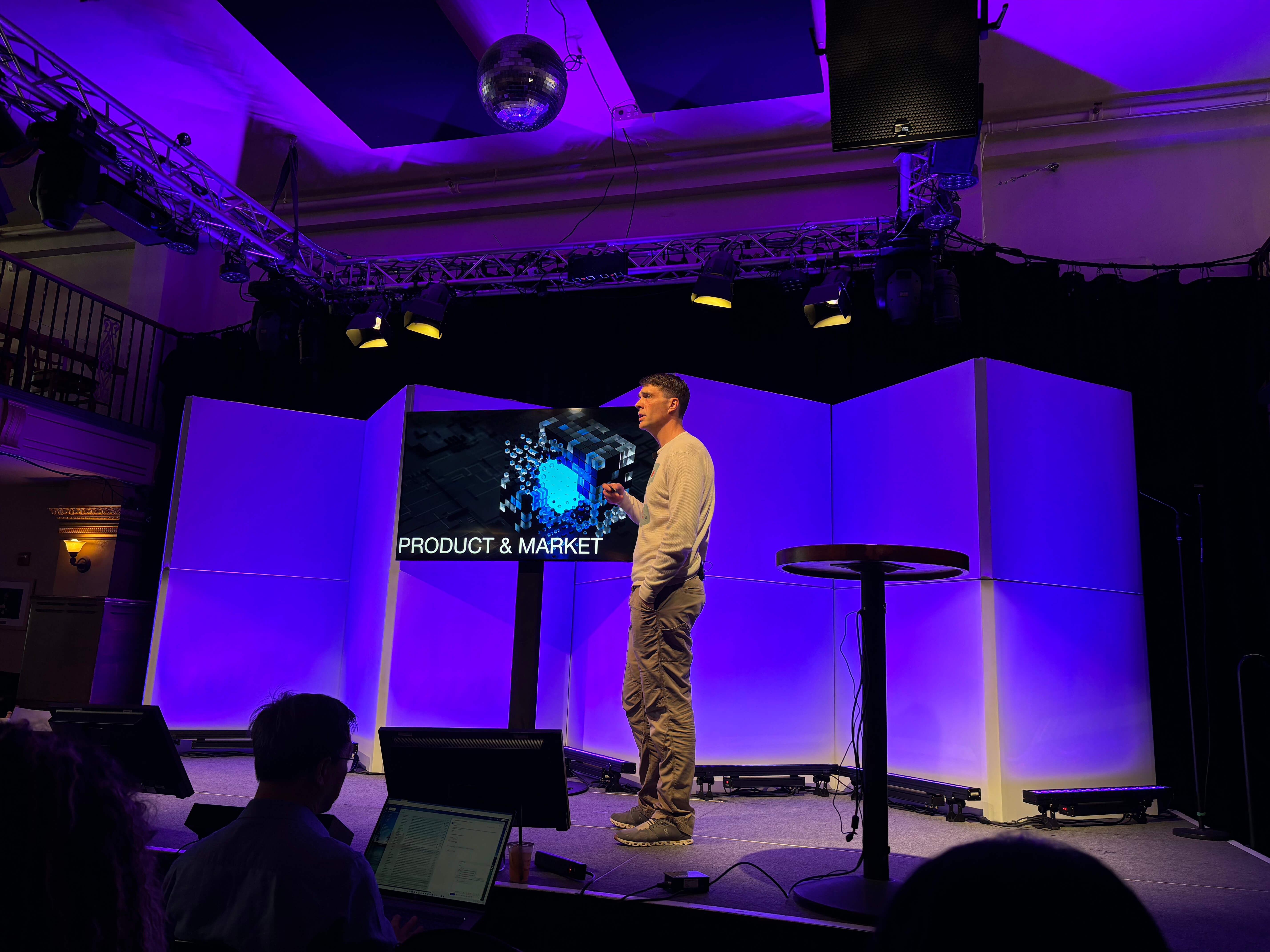
Agentic systems are often evaluated on how quickly they return outputs, but speed alone is a poor proxy for performance. If results arrive too late to inform a decision — or too early to be trusted without further revision — the system fails to deliver real value. The true measure of performance is time to value: how long it takes to produce an output that is not only accurate but also intelligible and actionable.
High latency and inconsistent response times prevent users from developing the familiarity and prompting precision needed for effective interaction. When outputs are slow or unpredictable, experimentation becomes costly and the feedback loop that drives fluency breaks down. This creates a structural barrier to adoption, especially in domains like investment research or financial due diligence where both timeliness and trust are critical.
Well-designed agentic systems address this by prioritizing both efficiency and interpretability. Rather than returning a single opaque answer, they break complex tasks into discrete steps, expose intermediate reasoning and provide structured outputs that support user oversight. This allows analysts to audit findings, apply their knowledge of domain-specific context and iterate alongside the AI platform as needed.
Systems that lack this transparency may appear capable in isolated demos, but without clear mechanisms for engagement and refinement, they are unlikely to perform reliably in real-world environments.
Change Management for Agents: Building AI-Native Teams
In organizations where AI agents drive core workflows, traditional team structures need to evolve. When agentic AI is capable of digesting documents, reasoning through mountains of data and surfacing initial cuts of insights, human teams can shift their time and analytical capacity from executing tasks to directing and validating the initial outputs of these systems.
This demands smaller, high-leverage teams that prioritize ownership, clarity of thinking and technical fluency. Analysts in these environments won’t need to dig through documents to find every data point by hand. Instead, they can act as strategic reviewers, applying historical or industry expertise to identify gaps and guide the system toward more relevant or nuanced analysis. The human value-add lies in an analyst’s ability to frame the problem, steer workflows and bring non-digitized insight into the decision-making process.
Rather than filling roles designed for throughput, AI-native organizations need individuals who can interpret model outputs, structure effective prompts and make context-sensitive decisions that incorporate both human judgment and machine reasoning.
An AI-native team is not simply smaller; it is structured for adaptability, focused on orchestrating decision processes and deeply integrated with the systems it works alongside.
The Private Market Case Study: Rethinking AI Due Diligence
Increasing competition for capital, a tighter fundraising environment, and macroeconomic volatility are increasingly putting pressure on private market teams to source more opportunities and make smarter bets in less time. In a traditional due diligence process, teams can be expected to review thousands of pages of data room documents, contracts, and expert call transcripts on compressed timelines, often with limited staffing. The result is a process strained by time, prone to error and highly dependent on human endurance.
Agentic systems can support the due diligence process by taking on the labor-intensive work of reading, extracting and identifying patterns across extensive document sets. These systems can aggregate findings from diverse sources, cross-reference them with historical deal data and organize the results into initial investment theses. They can also surface key no-go risk factors such as early termination clauses, inconsistent vendor statements and thematic exposure patterns early on in a diligence process.
This capability is not theoretical. In production environments, Brightwave as a deep research tool actively analyzes data rooms containing thousands of pages of documentation. Outputs are structured, context-aware and fully traceable to their original sources, allowing teams to audit conclusions and explore supporting evidence. At every step, humans remain in the driver’s seat, but are able to edit or validate Brightwave’s findings and reports rather than spending days or hours generating them manually.
By restructuring how diligence is performed, agentic systems reduce the risk of missed insights and increase both the speed and accuracy of evaluations. The result is faster deal execution combined with a higher-resolution understanding of risk and opportunity.
Final Take: AI Is Infrastructure, Not Magic
The most effective AI systems don’t behave like assistants or advisors. They function as infrastructure, built to absorb complexity, surface insights and support decision-making at scale. As the field evolves, the distinction between AI that simply executes and AI that supports multi-step reasoning over complex documents will become increasingly clear. The teams that design with this understanding, and the investors who recognize the difference, will help define what meaningful AI deployment looks like in practice.
Ready to Build Smarter?
Brightwave is helping investment teams think faster, gain conviction and unlock deeper insights with an AI platform built for real-world complexity.
Want to see how our systems work or talk about how AI can accelerate your process? Contact us or schedule a demo to start the conversation.